Hot topics – Reimagining data governance for AI
Key themes and emerging challenges: Safeguarding security and strengthening quality
When healthcare professionals (HCPs) are asked to identify barriers to introducing and scaling artificial intelligence (AI) in their sector, data is always raised as a major concern. Particular apprehensions include: data quality, security, and interoperability, in addition to the conundrum of who owns it.1 What’s more, healthcare is historically among the least digitised sectors in Europe, and this lack of high-quality digital records can mean there is insufficient data to ‘feed the algorithms’.1 Consequently, appropriate data governance for AI is crucial to overcome these challenges and successfully scale the technology in healthcare.
Data-hungry algorithms: the challenges
Large amounts of digitised data are required to develop, train and test AI algorithms. Although healthcare creates vast quantities of data, access is limited due to a lack of digitisation.1 Expert participants across the seven European Round Table Meetings agreed that a reliance on manual or paper records means a significant proportion of the data held by healthcare systems cannot be easily accessed for use with AI solutions. Moreover, reliance on manual processes means that there is rarely standardisation or interoperability of systems within, or between, care providers.1 This means clinical systems often function independently and records cannot communicate with each other – even information relating to an individual patient may not be fully integrated.1
[We need] enough data to train algorithms – while also preserving public trust, using regulation to ensure citizens feel protected, without stifling innovation.
Many of the participants across the seven Round Table Meetings suggested basic digitisation of systems and data within healthcare, supported by digital skills training for HCPs, is necessary for the adoption and scaling up of AI. However, building large linked data sets that are interoperable and can be accessed as needed will require big changes to workflow and workforce skills, as well as strong governance to ensure data security.1
Key insights – Netherlands
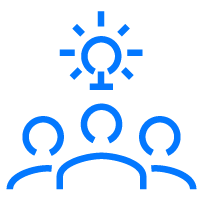
Data access is a fundamental requirement for the development and implementation of AI applications but it is an ongoing challenge in the Netherlands.
Investment is needed to standardise existing clinical data and make it suitable for AI use. This could be in the form of public funding with organisations charged for access.
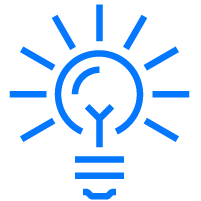
Participants at the Round Table Meeting referenced the FAIR Guiding Principles for scientific data.2* To support the development of FAIR databases, the GO FAIR Foundation was established in February 2018 as a separate legal entity under Dutch law.
GO FAIR and other partners were commissioned to develop a national COVID-19 data portal to help researchers to find and reuse COVID-19-related observational data from Dutch health care providers. This has included a governance policy for data sharing: researchers can gain access to FAIR research data while adhering to legal conditions and the privacy of patient’s data. The initiative is an example of what can be achieved when there is an urgent need.
*The FAIR Guiding Principles provide guidelines for creating databases that are Findable, Accessible, Interoperable and can be Reused2
Accessing healthcare data securely: the way forward
Participants at the Round Table Meetings highlighted that European healthcare organisations do have strong experience with data governance that they can draw on when adopting AI technology.
Healthcare systems are used to dealing with sensitive personal data securely, and existing European security laws should provide a degree of reassurance. Round Table Meeting participants suggested that high-profile cases of data security breaches (including failures to gain appropriate consent for access to data and lack of transparency around its uses), may cause some nervousness about introducing digital technologies in healthcare. However, the adaption of robust data-sharing policies would enable healthcare systems to benefit from AI while putting in place the appropriate safeguards.1
Further, health organisations already have experience communicating with each other, and hold large datasets that are shared within health systems. For example, the European Nucleotide Archive, and anonymised data from genome projects in Denmark and the UK are available to access for research purposes within strict guardrails. These experiences and processes should be used to inform and update existing governance when implementing AI.1
A typical hospital in Poland is reporting daily, to six databases. The question is – do these six databases talk to each other? Are they connected? Quite another thing is the reliability of reporting – daily reporting requirements are not always adhered to consistently across hospitals.
Key insights – Denmark
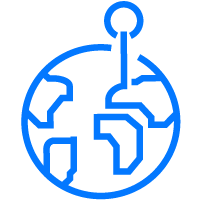
Round Table Meeting participants advised that Denmark already has a good reputation for quality and storage of data. But how can it go from ‘good’ to ‘excellent’?
Participants highlighted that new standards for data are not required and Denmark can learn from advances being made, such as the Fast Healthcare Interoperability Resources (FHIR) – the global standard for sharing healthcare data between systems.3
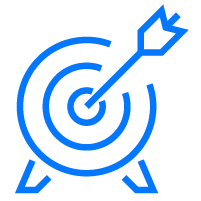
Denmark has many data banks, but lacks the infrastructure to improve the quality. Data-cleaning algorithms are not routinely shared, but should be.
Initiatives are underway, however, at a regional and national level. For example, the Radiological AI Test Centre, which has a data infrastructure and an anonymisation robot, is being established in the Capital Region of Denmark.
Europe also benefits from the General Data Protection Regulation (GDPR), which aims to protect personal data while allowing it to flow freely within the EU.1 GDPR has provided a new standard for privacy protection worldwide, and participants at the Round Table Meetings highlighted that Europe could take a similar leading role in developing data security governance in the AI sector.1 Indeed, participants advised that with GDPR as a basis, it is possible to begin creating processes and regulation that enable innovators to access data. Databases built on the FAIR Guiding Principle, and underpinned by GDPR compliance, could enable regulated access to data by companies looking to validate AI algorithms.2
In terms of next steps and recommendations, support is needed from the EU to provide guidance around data governance strategy, advised Round Table Meeting participants. Participants also recommended appointing national entities or institutions to act as guardians of health data. Whatever solutions are found to manage data governance, AI in healthcare is a fast-moving field and if Europe is to benefit from – and lead the way – in harnessing the technology’s benefits for its citizens, we must move fast to keep up.
Let us know your thoughts on optimal data governance in AI by tagging @EITHealth on Twitter or Facebook and using the hashtag #EITHealthAI.
References
1 EIT Health and McKinsey & Company. Transforming healthcare with AI: The impact on the workforce and organisations. 2020. Available from: https://eithealth.eu/wp-content/uploads/2020/03/EIT-Health-and-McKinsey_Transforming-Healthcare-with-AI.pdf (accessed January 2021).
2 Wilkinson MD, Dumontier M, Aalbersberg IJ, et al. The FAIR Guiding Principles for scientific data managementand stewardship. Sci Data 2016; 3: 160018. doi: 10.1038/sdata.2016.18.
3 FHIR Overview. Available from: https://www.hl7.org/fhir/overview.html (accessed January 2021).