Hot topics – Navigating liability and managing risk in AI
Key themes and emerging challenges: who is accountable for AI and how can we gain trust?
Accountability within the healthcare sector is paramount for patient safety, as well as the professional liability of clinicians.1 This can lead to healthcare systems that are risk averse, which can be a barrier to changing current practices.1–3 The issue of liability for AI applications is a particular challenge, with the hospital, healthcare professional (HCP) or algorithm trainer all potentially accountable.1 Furthermore, it remains unclear how risk and liability can be effectively monitored over the lifecycle of an AI application, particularly if the AI changes its behaviour over time.
What might all this mean for the large-scale adoption of AI in the healthcare sector?
Assigning responsibility
Liability and managing risk were tabled for discussion at the seven Round Table Meetings, and discussions highlighted that in the current European landscape, accountability for AI is opaque. Part of the difficulty in assigning responsibility stems from the distinction between AI as a decision-support tool and AI as a decision-making tool.1 Many AI applications already being used in hospitals enhance or support a doctor or nurse in making clinical decisions, in which case the HCP ultimately decides and remains responsible for incorrect use and the consequences of any subsequent decisions.1 However, matters will be less clear cut as AI becomes more autonomous and capable of automated decision-making. At this point, deciding where the professional responsibility begins and ends will be key to widespread adoption of AI in healthcare.1
Participants across the Round Table Meetings considered that new roles may be needed to assume responsibility and monitor risk and liability within healthcare systems adopting AI. In particular, hiring a chief information officer (CIO) for AI recognises that deployment of such technology requires dedicated time and expertise. Moreover, AI requires large amounts of data, making data security a concern. Whilst healthcare systems are typically used to handling large amounts of data, participants highlighted that a CIO could be a useful support by overseeing all data processing as well as permissions for data use.1
Key insights – Germany
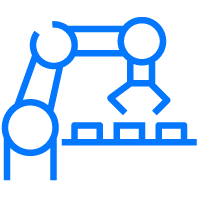
The Round Table Meeting participants in Germany focused on the distinction between AI solutions that run autonomously and those which, for example, support medical decision-making in the background.
Participants shared that with the exception of a single ophthalmological application, autonomous AI systems have not yet been used in the German healthcare system. A widespread introduction of these systems is not expected in the near future – when that does happen regulation will be required to manage liability issues.
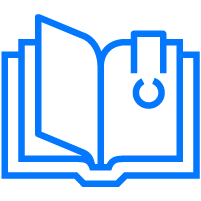
It is unclear if clinicians would be liable if they follow an incorrect recommendation from an AI solution. However, there was broad agreement amongst the Round Table Meeting participants that AI-based systems reduce the overall error rate in clinical decision-making, and therefore the potential benefits of their use outweigh the risk.
In Germany, an overarching legal regulation is expected to regulate liability issues. This would include other sectors as well as healthcare e.g., the motor industry and the use of self-driving cars. In this area, initially the focus will be on collecting real-world data in order to calculate the insurability of the risks of AI.
Regulation is needed to manage risk
While important, managing risk and liability for AI in healthcare is about more than assigning roles and responsibilities. Effective processes and regulation must also be in place to support those held accountable.1 Core to this is transparency and the development of explainable AI, advised participants across the Round Table Meetings.4,5 For regulators, as well as care teams implementing AI, being able to understand how a particular AI solution generates its outputs may be key to reducing risk, gaining trust, and determining who will be responsible if it should ‘fail’.6
Effective risk management of AI in healthcare will also require regulators to develop international standards for designing and monitoring AI.5 Regulation was discussed in depth at the Round Table Meetings and participants discussed the need for regulation to recognise risk at several points in the AI lifecycle, including:
- Data quality during initial development
- The potential consequences of using AI in environments that are different to that in which the AI was developed
- The possible changes to outputs that might occur as some AI algorithms learn and update over time (adaptive algorithms).5
While fixed algorithms that do not change over time might fit well within current medical device regulations, adaptive algorithms do not.7 It is therefore possible that a one-size-fits-all regulatory model may not be appropriate for AI,5 and new policies and regulatory processes for AI solutions may be required.1
Furthermore, all AI solutions require large amounts of data and risk is associated with this. Regulation to ensure data security will be a critical issue in terms of risk management if AI is to be rolled out across healthcare systems.1 Standards to ensure data is anonymous and secure, as well as identifying who is responsible for monitoring and enforcing these standards is needed to ensure the implementation of AI in healthcare is ethical, technically robust and lawful.1
Key insights – Spain
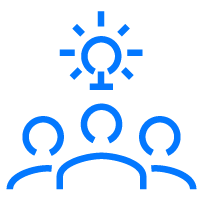
The key is to successful AI implementation in healthcare is confidence – only a system that generates confidence will end up being regulated and adopted. The algorithm can support and make recommendations, but the medical professional must make the decisions.
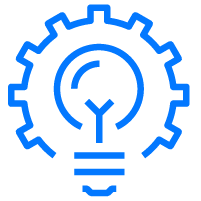
Adaptive AI may advance to the point where it can draw correlations between information and data that is unknown to the programmer / user of the AI system. Management of this must be supported by periodic monitoring of the algorithms.
The unanswered questions regarding accountability and liability in AI are complex and wide-ranging. Participants across the Round Tables Meetings advised that the use of all new technologies carries an element of risk, and AI is no exception. However, effective ways to manage this risk are emerging and these solutions will be key to progressing and enabling successful AI adoption across European healthcare systems.1
What do you think is the biggest risk posed by adoption of AI in healthcare and how can we mitigate it? Share your ideas and thoughts on our Twitter and Facebook channels, @EITHealth, using the hashtag #EITHealthAI.
References
1 EIT Health and McKinsey & Company. Transforming healthcare with AI: The impact on the workforce and organisations. 2020. Available from: https://eithealth.eu/wp-content/uploads/2020/03/EIT-Health-and-McKinsey_Transforming-Healthcare-with-AI.pdf (accessed January 2021).
2 The Health Foundation. What’s getting in the way? Barriers to improvement in the NHS. 2015. Available from: https://www.health.org.uk/sites/default/files/WhatsGettingInTheWayBarriersToImprovementInTheNHS.pdf (accessed January 2021).
3 Eichler HG, Bloechl-Daum B, Brasseur D, et al. The risks of risk aversion in drug regulation. Nature Rev Drug Discov 2013; 12(12): 907–916.
4 Gerke S, Minssen T, Cohen G. Ethical and legal challenges of artificial intelligence-driven healthcare. Artificial Intelligence in Healthcare 2020. DOI: 10.1016/B978-0-12-818438-7.00012-5.
5 Cohen IG, Evgeniou T, Gerke S, Minssen T. The European artificial intelligence strategy: implications and challenges for digital health. Lancet Digital Health 2020; 2: e376–e379.
6 Kuan R. Adopting AI in Health Care Will Be Slow and Difficult. 2019. Harvard Business Review. Available from: https://hbr.org/2019/10/adopting-ai-in-health-care-will-be-slow-and-difficult (accessed January 2021).
7 PHG Foundation. Algorithms as medical devices. 2019. Available from: https://www.phgfoundation.org/documents/algorithms-as-medical-devices.pdf (accessed January 2021).